Neural Network 简介 学习笔记
2023-1-6|2024-4-11
Pat
type
status
date
slug
summary
tags
category
icon
password
神经网络
神经网络中的节点为neuron。上下层之间neuron的参数为weight和bias。不同层之间的计算可以用矩阵表示,下一层激发值的计算可以用Linear algebra 表示。

其中 函数为一个投影函数,目的是将激发值投射在0-1。Sigmoid是S型函数,现代中更常使用ReLu函数作为 函数。


Training and Back-propagation
为了得到满意的结果(恰当的激发值),我们需要将神经网络进行训练,即找到接近最佳的weight和bias参数。
当输入一个训练样本时(即样本已经被正确tag好),得到目前神经网络的Output,当然未经妥善训练过的神经网络的Output会与我们期望的正确结果有偏差,我们开始思考如何修改weight和bias参数可以使结果更接近正确。由最后一层的 反推前面层的weight和bias参数和 的改变,甚至前面一层的 等,这就是Back-propagation。
Cost Function- A way to evaluate the network
首先,构造一个Cost function。
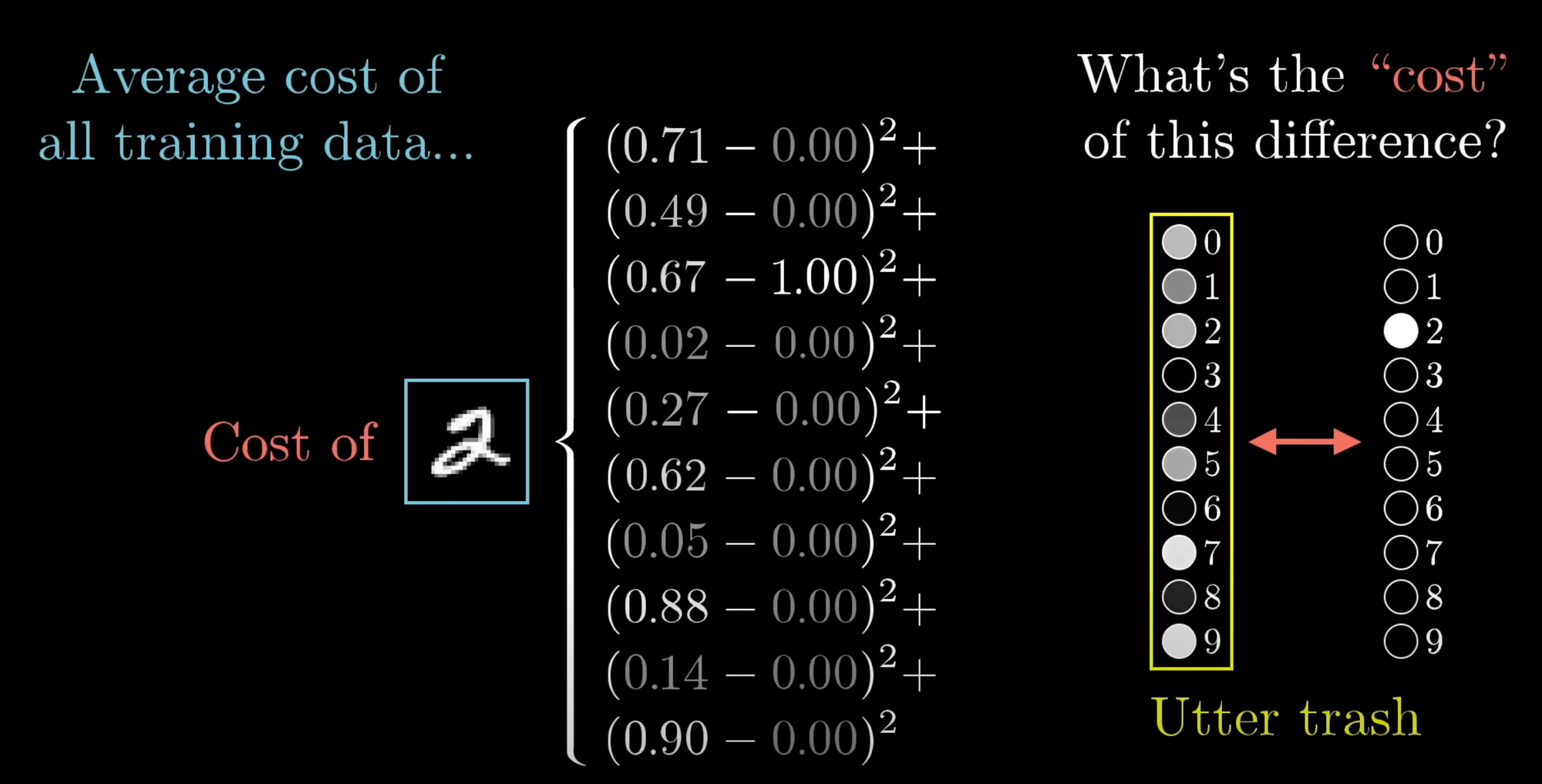
在这个例子中Cost Function为Last Layer Output 与 Expected Output的方差的和。
模型充分训练,由Cost Function更小给出,Cost function更小即输出结果与我们Expected Output value 更接近。
Which way to change to direction of factor?——Gradient descendant
如何使模型训练更好,参数更准确的问题转换为求Cost Function的最小值的数学问题。
将Input视为一个Vector,Training的问题就转变为Vector在Model中得到最小的 ,Gradient descendant给出了Minimum Cost Function的路径。
Cost Function对Factor求偏导,根据链式法则:
每个偏导的符号和大小分别指明factor需要改变的方向和改变的程度。
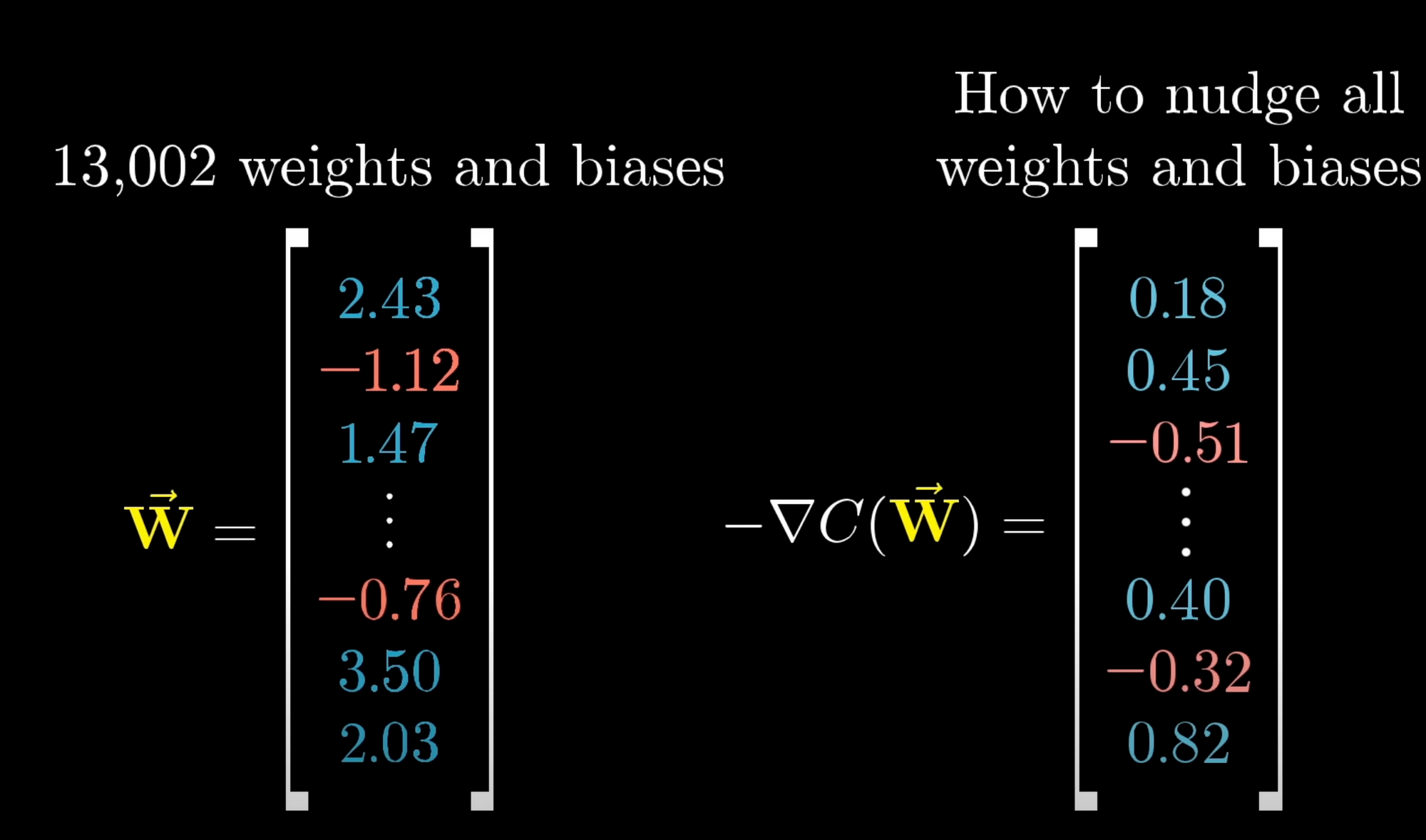
(附:我们想要minimum ,因此改变方向与导数方向相反)
Gradient decendant
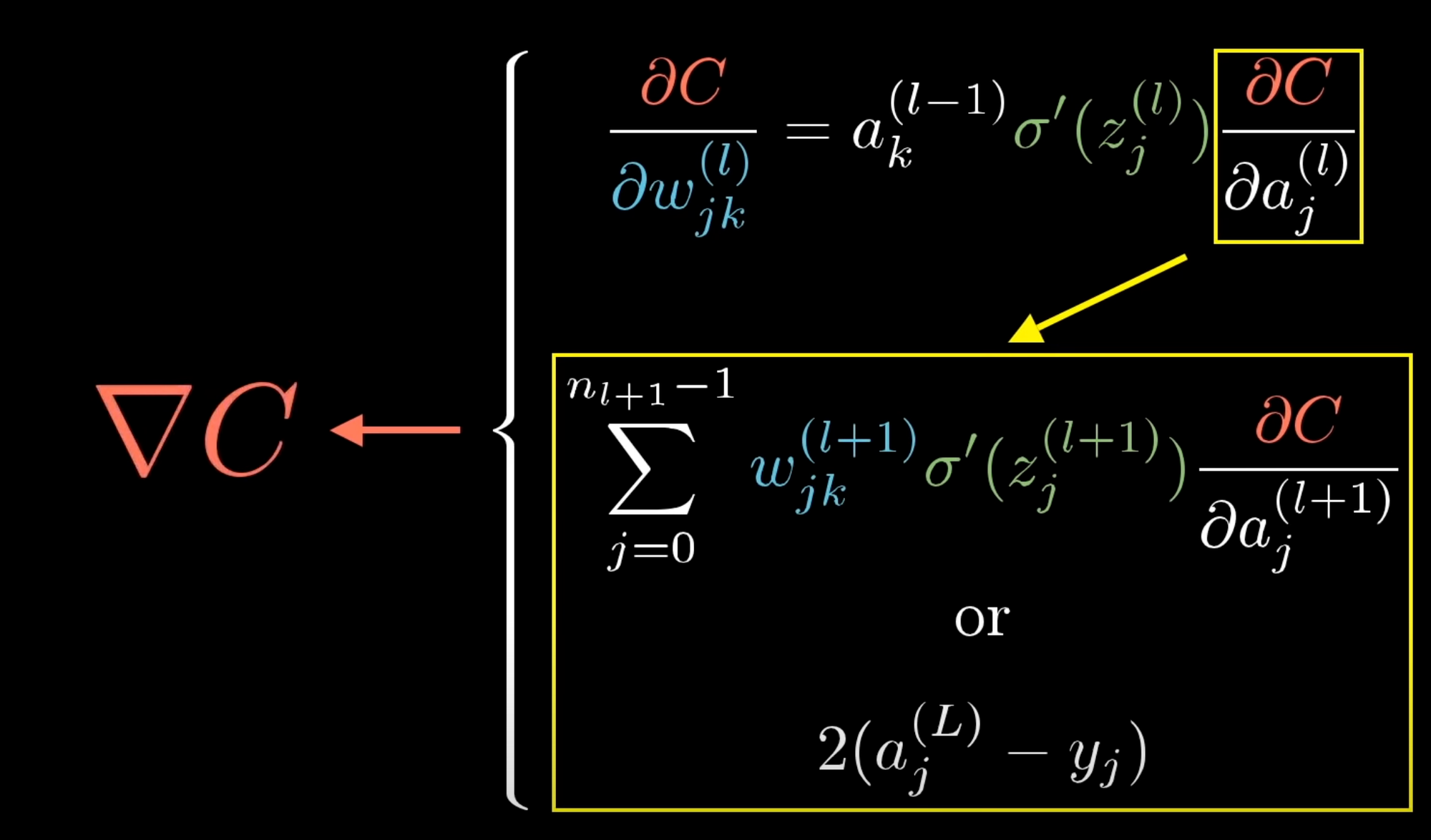
Source:
Loading...